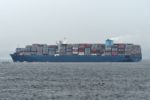
Analyst Insight: At its core, artificial intelligence is about trust. If humans don’t trust their technology, they won’t use it. As many organizations struggle to achieve full value realization, the importance placed on trust in the user-AI relationship continues to grow. This is particularly crucial in supply chains, where AI could promise optimized inventories, predictive maintenance, streamlined supplier risk management and better route optimization. The challenge? Establishing trust to drive AI adoption and maximize its time to value.
When users trust AI, they integrate its insights more deeply into their workflows, leading to more effective outcomes. By contrast, a lack of trust can negate even the most advanced supply chain AI models, which may lead users to revert to less efficient, traditional methods.
Central to fostering this trust is the active construction of a symbiotic relationship between AI and its users, a process that can be based on the four factors of trust: Capability, Reliability, Transparency, and Humanity.
Capability refers to the accuracy of the AI model in performing designated tasks, such as predicting inventory needs or identifying potential equipment failures. This can be quantified through measures like recall, a metric that assesses the model's aptitude in capturing all relevant instances. For example, an AI model tasked with predicting inventory shortages but exhibiting low recall would miss numerous actual shortages. This shortfall in performance undermines trust, as users depend on its insights to make timely, informed decisions that optimize supply chain operations.
Reliability refers to the consistency of the AI model’s predictions and recommendations. This can be quantified through the measure of precision, a metric that evaluates the model’s ability to make correct predictions. Consider an AI model designed to identify defective products in a shipment; in this context, high precision means it accurately identifies defective items without producing false positives. Accomplishing this involves a rigorous approach to AI deployment, typically anchored by machine learning. Reliability is what transforms AI from a mere tool into a trusted partner.
Transparency in AI involves the explainability of the AI model and transparency of its implementation. An explainable AI model clearly articulates how its recommendations are derived, fostering user understanding and trust in the underlying logic. Moreover, providing an understanding behind the AI deployment reassures users that the technology aims to augment their capabilities.
Humanity in AI implementation is crucial, especially in supply chain contexts where AI impacts ways of working. This involves creating AI systems that are ethical and fair, ensuring a balanced integration of technology in the workplace. A human-centric approach in AI is essential for positive AI applications and outcomes. Incorporating supply chain analysts and inventory managers in creating an AI model for demand forecasting, for example, helps users understand and trust the logic behind the AI’s operations, while encouraging its adoption and integration into decision-making processes. It also diminishes the "black box" effect that can potentially lead to low user engagement.
Outlook: Unequivocally, trust in AI is a cornerstone for transformative success. It’s the bridge between AI’s potential and its real-world application. As supply chains evolve, embracing AI with confidence is key to unlocking efficiencies, predicting trends, and navigating complex networks. Trust isn’t just a factor; it’s the foundation upon which the future of streamlined intelligent supply chains will be built.
Resource Link:
RELATED CONTENT
RELATED VIDEOS
Timely, incisive articles delivered directly to your inbox.